“Fine Land Cover Classification in an Open Pit Mining Area Using Optimized Support Vector Machine and WorldView-3 Imagery” is published in Remote Sensing, 2020, 12(1), 82. It is an achievement of associate professor CHEN Weitao’s team from the School of Computer Science. CHEN is the first author, and the corresponding author is associate professor LI Xianju.
Fine land cover classification in an open pit mining area (LCCOM) is essential in analyzing the terrestrial environment. However, researchers have been focusing on obtaining coarse LCCOM while using high spatial resolution remote sensing data and machine learning algorithms. Although support vector machines (SVM) have been successfully used in the remote sensing community, achieving a high classification accuracy of fine LCCOM using SVM remains difficult because of two factors. One is the lack of significant features for efficiently describing unique terrestrial characteristics of open pit mining areas and another is the lack of an optimized strategy to obtain suitable SVM parameters. This study attempted to address these two issues. Firstly, a novel carbonate index that was based on WorldView-3 was proposed and introduced into the used feature set. Additionally, three optimization methods—genetic algorithm (GA), k-fold cross validation (CV), and particle swarm optimization (PSO)—were used for obtaining the optimization parameters of SVM. The results show that the carbonate index was effective for distinguishing the dumping ground from other open pit mining lands. Furthermore, the three optimization methods could significantly increase the overall classification accuracy (OA) of the fine LCCOM by 8.40%. CV significantly outperformed GA and PSO, and GA performed slightly better than PSO. CV was more suitable for most of the fine land cover types of crop land, and PSO for road and open pit mining lands. The results of an independent test set revealed that the optimized SVM models achieved significant improvements, with an average of 8.29%. Overall, the proposed strategy was effective for fine LCCOM.
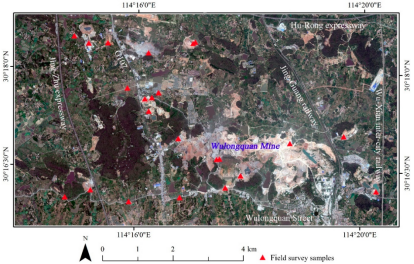
Figure 1. Study area and WV-3 fused true color image. RGB = band 5, 3, 2.
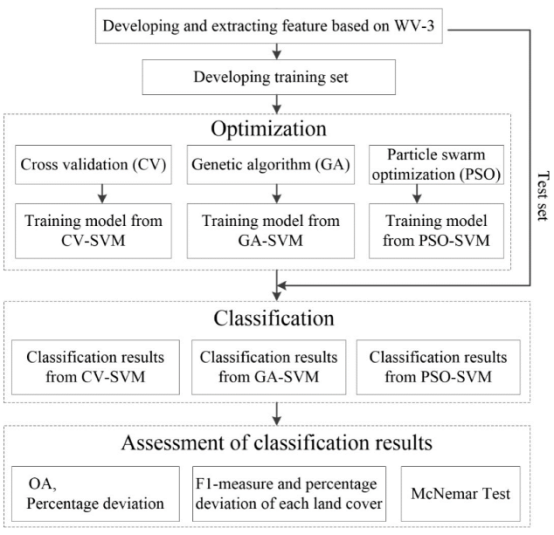
Figure 2. Overall flowchart of the methodology employed in this study.
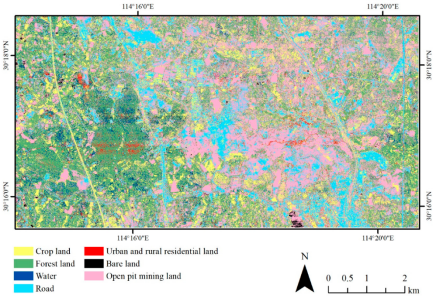
Figure 3. The predicted map of the study area with the first-level land covers.
Full Text: https://www.mdpi.com/2072-4292/12/1/82